20 Top Facts To Deciding On AI Stock Predictions Analysis Websites
Top 10 Tips For Evaluating The Ai And Machine Learning Models Of Ai Stock Predicting/Analyzing Trading PlatformsAssessing the AI and machine learning (ML) models utilized by trading and stock prediction platforms is vital in order to ensure that they are accurate, reliable, and useful insights. Models that are not designed properly or overhyped could lead to inaccurate predictions and financial loss. Here are 10 of the best ways to evaluate the AI/ML model used by these platforms.
1. The model's purpose and approach
Determining the objective is important. Make sure the model was designed to be used for long-term investment or for trading on a short-term basis.
Algorithm transparency: Make sure that the platform discloses the types of algorithms employed (e.g., regression or neural networks, decision trees or reinforcement learning).
Customization. Determine whether the model is able to be modified according to your trading strategy, or your risk tolerance.
2. Review Model Performance Metrics
Accuracy: Test the accuracy of the model when it comes to predicting future events. However, do not solely depend on this measurement since it can be inaccurate when applied to financial markets.
Accuracy and recall: Check whether the model is able to discern real positives, e.g. correctly predicted price changes.
Risk-adjusted return: Determine whether the model's predictions result in profitable trades after accounting for risks (e.g. Sharpe ratio, Sortino coefficient).
3. Test the Model by Backtesting it
Backtesting your model with the data from the past allows you to test its performance against prior market conditions.
Out-of sample testing The model should be tested using data it wasn't trained on in order to avoid overfitting.
Analysis of scenarios: Check the model's performance during different market conditions (e.g., bull markets, bear markets, high volatility).
4. Check for Overfitting
Signs of overfitting: Search for models that perform exceptionally good on training data but struggle with data that isn't seen.
Regularization methods: Check the application uses techniques such as L1/L2 regularization or dropout to avoid overfitting.
Cross-validation: Ensure that the model is cross-validated in order to evaluate the generalizability of the model.
5. Examine Feature Engineering
Relevant features: Check if the model uses relevant features (e.g. volume, price sentiment data, technical indicators macroeconomic variables).
Selection of features: Make sure that the system chooses characteristics that have statistical significance. Also, do not include irrelevant or redundant data.
Updates to dynamic features: Determine whether the model adapts in time to new features or changes in market conditions.
6. Evaluate Model Explainability
Interpretability - Make sure that the model offers an explanation (e.g. values of SHAP or the importance of a feature) to support its claims.
Black-box models cannot be explained Be wary of software using overly complex models, such as deep neural networks.
User-friendly insights: Ensure that the platform offers actionable insights that are presented in a manner that traders are able to comprehend.
7. Examining Model Adaptability
Market conditions change - Check that the model is modified to reflect changes in market conditions.
Check for continuous learning. The platform should be updated the model frequently with new data.
Feedback loops - Ensure that the platform is able to incorporate real-world feedback and user feedback to improve the design.
8. Check for Bias in the Elections
Data bias: Make sure that the data regarding training are representative of the market, and free of bias (e.g. overrepresentation in certain time periods or sectors).
Model bias: Determine if you are able to actively detect and reduce biases that exist in the predictions of the model.
Fairness: Ensure that the model doesn't unfairly favor or disadvantage particular sectors, stocks, or trading styles.
9. Examine Computational Efficiency
Speed: Determine whether a model is able to make predictions in real time with the least latency.
Scalability - Ensure that the platform can manage massive datasets, multiple users, and does not affect performance.
Resource usage : Determine if the model has been optimized to use computational resources effectively (e.g. GPU/TPU).
Review Transparency and Accountability
Model documentation: Verify that the platform provides complete documentation about the model's design, the process of training and its limitations.
Third-party audits : Confirm that your model was audited and validated independently by a third party.
Make sure there are systems that can detect mistakes and failures of models.
Bonus Tips:
User reviews and case studies User feedback and case studies to gauge the performance in real-life situations of the model.
Trial period: You can use a free trial or demo to evaluate the model's predictions as well as its usability.
Customer support: Ensure your platform has a robust support for model or technical problems.
These suggestions will assist you to examine the AI and machine-learning models used by platforms for prediction of stocks to ensure they are transparent, reliable and aligned with your goals for trading. Have a look at the best ai for investing recommendations for site tips including ai investing, best ai trading app, ai for stock trading, options ai, best ai stock trading bot free, ai stock trading bot free, ai for stock predictions, best ai trading app, best ai trading app, best ai stock trading bot free and more.
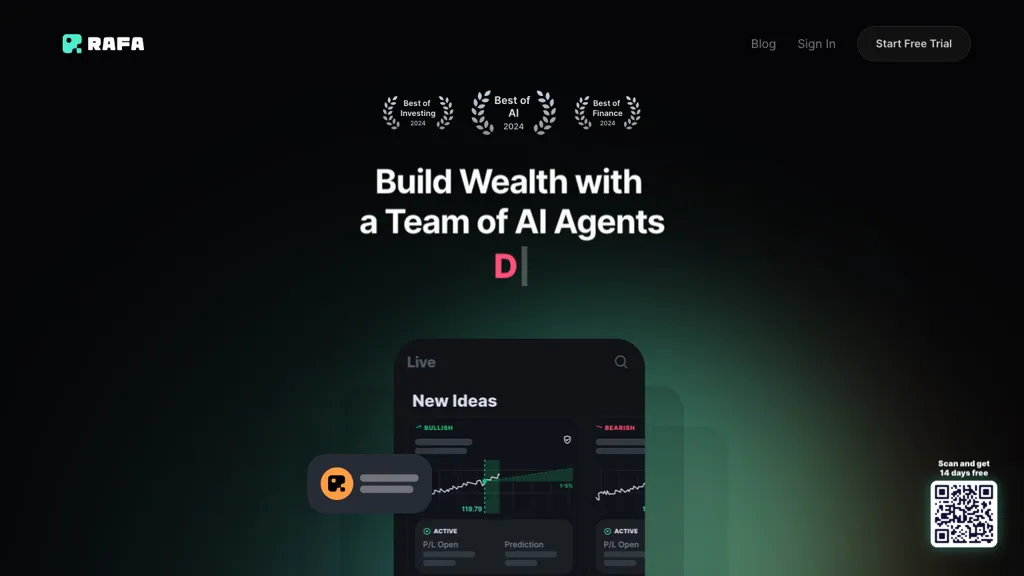
Top 10 Tips To Assess The Updates And Maintenance Of Ai Stock Trading Platforms
It is crucial to evaluate the maintenance and updates of AI-driven trading and stock prediction platforms. This will help ensure that they are safe and in line with changing market conditions. Here are 10 best suggestions for evaluating their updating and maintenance methods.
1. The frequency of updates
Check out the frequency at which updates are released (e.g. every week, each month, or every quarter).
Regular updates show the ongoing development of the product and a willingness to respond to market changes.
2. Transparency in Release Notes
Tips: Read the release notes on your platform to learn about any improvements or modifications.
Why: Transparent Release Notes demonstrate the platform's dedication to continual improvement.
3. AI Model Retraining Schedule
Tips: Find out how often the AI models are trained using new data.
Why: Models must evolve to remain relevant and accurate as markets shift.
4. Bug Fixes and Issue Resolution
Tips: Find out how quickly the platform responds to bugs or issues that users report.
The reason: Quick bug fixes ensure the platform's reliability and operational.
5. Updates on Security
Tip: Verify if the platform regularly updates its security protocols to protect user data and trading activities.
Why is cyber security important? for financial platforms, as it helps stop fraud.
6. Integration of New Features
Examine the platform to determine if it has added new features in response to market or user feedback (e.g. improved analytics).
Why: The feature updates demonstrate the ability to innovate and respond to the needs of users.
7. Backward Compatibility
Make sure that any the updates won't affect existing functionality, or require significant reconfiguration.
What is the reason? Backward compatibility guarantees an enjoyable user experience during transitions.
8. Communication with users during maintenance
Tip: Find out how users are informed of planned maintenance or downtime.
Clare communication reduces disruptions and builds confidence.
9. Performance Monitoring and Optimization
TIP: Make sure the platform continuously monitors the performance metrics (e.g. accuracy, latency) and improves the performance of systems.
The reason is that ongoing optimization can ensure that the platform stays efficient.
10. Compliance with Regulatory Changes
TIP: Check if the platform offers new features or policies that comply with regulations governing financial transactions and data privacy laws.
Why: To avoid legal risk and maintain user's confidence, compliance with the regulatory framework is crucial.
Bonus Tip: User Feedback Integration
Make sure that the platform is actively incorporating user feedback into maintenance and updates. This indicates a strategy that is based on user feedback and a desire to improve.
Through analyzing all these factors, it is possible to ensure that you are sure that the AI stock trading platform you select has been maintained properly. It must also be up-to-date and adaptable to the changing dynamics of markets. Have a look at the top rated trading ai tool for blog recommendations including chart ai trading, ai for trading stocks, can ai predict stock market, best ai for stock trading, ai investment tools, can ai predict stock market, ai tools for trading, invest ai, stock predictor, investing with ai and more.
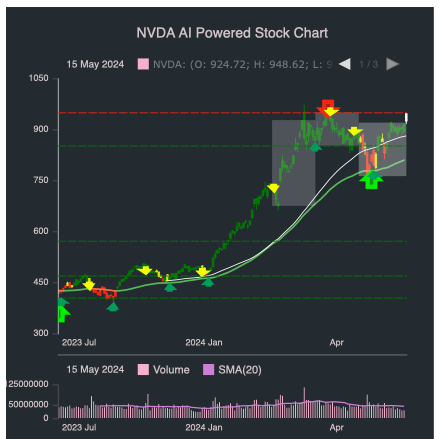